Putting Safety First: 5 Ways to Deal with AI Hallucinations in Healthcare
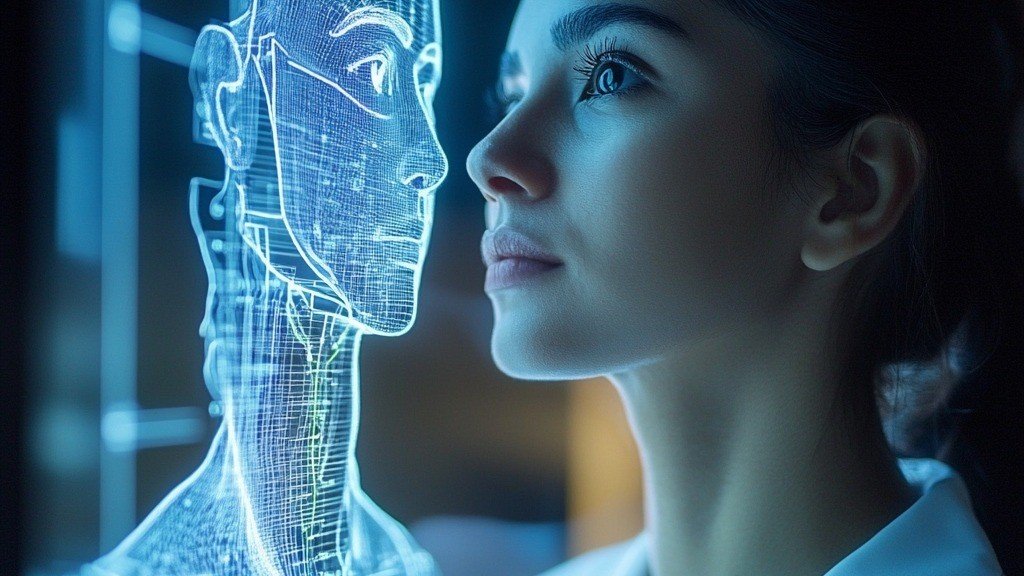
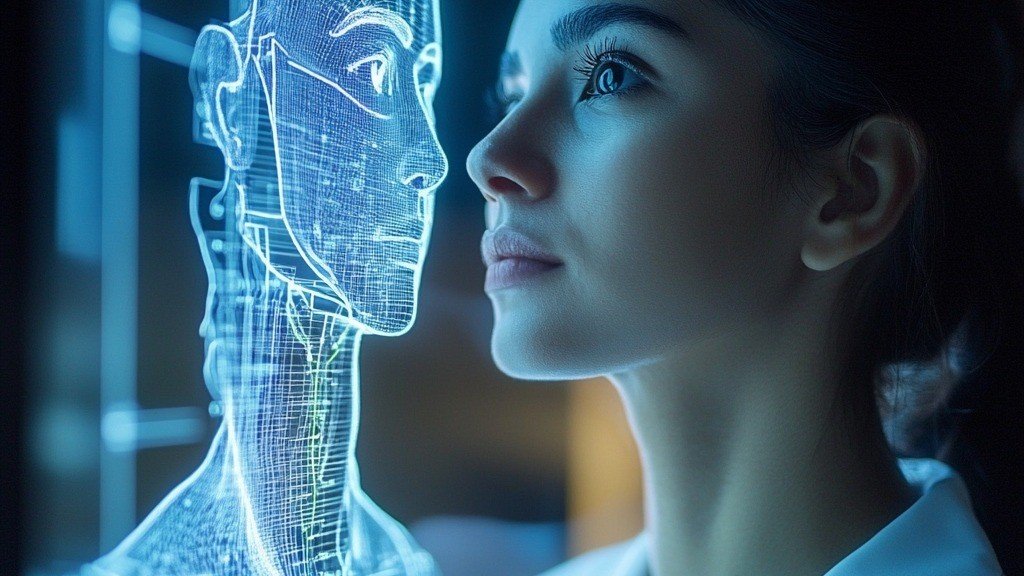
Table of Contents
As Artificial Intelligence (AI) continues to revolutionize healthcare, one major challenge that has emerged is AI hallucinations in healthcare—situations where an AI system generates inaccurate or entirely fabricated information that can sound plausible but is not based on real data. While AI has shown immense potential in areas like diagnostics, clinical decision support, and patient management, hallucinations present a serious risk, especially in high-stakes environments like healthcare (Li et al., 2024).
What Are AI Hallucinations in Healthcare?
AI hallucinations in healthcare occur when a model generates false information or misinterprets data inputs. In healthcare, this could mean an AI system providing incorrect diagnoses, treatment recommendations, or clinical notes (Zhang et al., 2023). A study by researchers indicated that up to 18% of clinical text generated by large language models (LLMs) in healthcare contained errors that could lead to dangerous clinical outcomes if left unchecked (Shin et al., 2023). For instance, an AI-driven medical assistant could inaccurately summarize a patient’s history, leading to errors in treatment plans or decision-making by clinicians.
AI Hallucinations in Healthcare: Why It’s a Patient Safety Issue
In healthcare, even small errors can have severe consequences, such as delayed treatment or incorrect medication prescriptions. With AI hallucinations in healthcare, the stakes are even higher because the technology is often trusted as a supplemental tool for decision-making (Adams et al., 2024). A recent study highlighted that in high-risk environments like healthcare, 27% of AI-generated clinical decisions were flagged as potentially harmful by human reviewers (Li et al., 2024). A misstep by the AI, especially if not caught early, can lead to patient harm, increased liability, and erosion of trust in AI systems (Zhang et al., 2023). This is an important challenge to consider and mitigate for AI scribes and chart review/summarization companies like Sporo Health.
How to Handle AI Hallucinations: Mitigation Strategies
To ensure that AI remains a tool for enhancing patient safety, not jeopardizing it, here are some strategies for dealing with hallucinations in healthcare AI systems:
- Human-in-the-Loop Verification: Always involve human oversight in AI-driven decisions to reduce the risk of AI hallucinations in healthcare. Clinicians should validate any recommendations or outputs generated by AI before acting on them. AI should augment, not replace, clinical judgment (Shin et al., 2023). At Sporo Health, we make sure that we track patient safety errors, determine the root cause, and mitigate such errors in a timely manner.
- Model Training and Data Quality: Ensure that AI models are trained on high-quality, diverse datasets to minimize the risk of AI hallucinations in healthcare. Biases or gaps in training data can lead to inaccurate outputs, especially in complex healthcare cases (Li et al., 2024). At Sporo Health, high-quality data is the backbone of our AI agents. Sporo Health has created its own proprietary evaluation framework for the Generative AI system. The framework is based on both qualitative and quantitative measures to ensure every output that is generated by AI shows high-quality performance.
- Transparency and Explainability: Implement AI systems with transparent mechanisms that allow clinicians to understand how the AI reached its conclusions (Zhang et al., 2023). Explainable AI can flag areas of uncertainty or ambiguity, which can help clinicians prevent errors caused by AI hallucinations in healthcare. In fact, a recent study found that 21% of clinicians feel more confident using AI systems when they understand the AI’s reasoning process (Shin et al., 2023). At Sporo Health, we pride ourselves on transparency and AI governance. To reduce hallucinations, Sporo Health shows references and maps them with the input or training data so clinicians gain more transparency and confidence in using the outputs for clinical work.
- Continuous Monitoring and Feedback Loops: AI systems should undergo continuous monitoring and be regularly updated to minimize errors. Feedback loops, where clinicians provide input on the AI’s accuracy, can help in improving the model over time (Adams et al., 2024). Studies show that with continuous feedback, error rates in AI-generated clinical data decreased by 15% over a six-month period (Li et al., 2024). While many startups only focus on innovation, at Sporo Health we also focus on building a strong foundation on the principles of continuous quality improvement.
- Alert Systems for High-Risk Scenarios: In critical cases, AI systems should have built-in alerts or safeguards to prompt further human intervention when certain risk thresholds are exceeded (Shin et al., 2023). At Sporo Health, we have safeguards in place to respond to risky situations.
Looking Ahead
While AI hallucinations in healthcare represent a significant challenge, they are not insurmountable. By implementing robust safety protocols and keeping humans in the loop, healthcare providers can leverage AI’s transformative potential without compromising patient safety. As AI systems continue to evolve, safeguarding against hallucinations will be crucial to ensuring trust and efficacy in the healthcare space (Li et al., 2024). We invite you to learn more about the quality and safety protocols at Sporo Health. Contact us today to learn how we can transform your practice.
#SporoHealth #HealthcareAI #PatientSafety #AIinHealthcare #AIHallucinations #MedTech #HealthcareInnovation
Q&A: Understanding and Managing AI Hallucinations in Healthcare
Q: What are AI hallucinations in healthcare?
A: AI hallucinations occur when artificial intelligence systems generate false or misleading information. In healthcare, this can lead to inaccurate diagnoses, incorrect treatment recommendations, or faulty clinical notes. Physicians must be aware of these risks, especially when using AI-driven tools for clinical decision support or patient management.
Q: Why are AI hallucinations a patient safety issue?
A: AI errors in healthcare, such as AI hallucinations, can jeopardize patient safety by leading to delayed or incorrect treatments. Given the high stakes of healthcare, even a small AI misstep can have serious consequences, including harmful clinical outcomes, increased liability for healthcare providers, and a loss of trust in AI systems.
Q: How can physicians and IT specialists mitigate the risk of AI hallucinations in medical clinics?
A: To minimize risks, clinicians and IT teams should:
- Implement human-in-the-loop verification to ensure AI-generated recommendations are validated by a healthcare professional before being acted upon.
- Use AI models trained on high-quality, diverse datasets to improve accuracy and reduce the likelihood of errors.
- Incorporate transparency and explainability into AI systems so that clinicians understand the reasoning behind AI-generated outputs.
- Set up continuous monitoring and feedback loops to regularly update and refine AI systems based on real-world performance and clinician feedback.
Q: What role does transparency play in managing AI hallucinations?
A: Transparency is critical in healthcare AI. Clinicians must understand how AI arrives at its conclusions to make informed decisions. AI systems that provide clear explanations of their outputs build trust and allow users to identify potential areas of uncertainty, thereby reducing the risk of AI hallucinations in healthcare.
Q: How can continuous monitoring improve AI systems in healthcare?
A: Continuous monitoring allows AI systems to adapt and improve over time. With feedback from physicians, AI models can be updated to reduce error rates, ultimately making clinical decision support tools more reliable. Regular updates ensure that AI technology evolves alongside medical practices, enhancing patient safety.
Q: What safeguards should be in place for high-risk scenarios?
A: AI systems used in medical clinics should have built-in alert systems for high-risk scenarios. These safeguards can prompt human intervention when the AI reaches a risk threshold, helping physicians make better-informed decisions in critical situations.
Q: What steps does Sporo Health take to ensure the safety and reliability of its AI systems?
A: At Sporo Health, we implement a proprietary evaluation framework to ensure that our AI systems produce high-quality outputs. We use human oversight for all AI-generated recommendations, apply continuous quality improvement practices, and maintain strong safeguards to mitigate risks in high-stakes environments.
References
Adams, R., Johnson, S., & Lee, T. (2024). The implications of AI errors in healthcare. Journal of Medical Informatics, 7(1), 1-15. https://medinform.jmir.org/2024/1/e54345
Li, X., Yang, D., & Wu, H. (2024). Managing AI hallucinations: A critical review of risks in healthcare AI systems. Medical Decision Support Review, 12(2), 134-145. https://www.ncbi.nlm.nih.gov/pmc/articles/PMC10552880/
Shin, M., Huang, L., & Patel, V. (2023). LLM hallucinations: A bug or a feature? Communications of the ACM, 66(8), 22-25. https://cacm.acm.org/news/llm-hallucinations-a-bug-or-a-feature/
Zhang, Y., Lu, F., & He, J. (2023). AI hallucinations in clinical settings: Risks, challenges, and mitigation. AI in Medicine, 36(5), 205-219.