6 Proven Strategies to Eliminate Bias in Clinical AI Scribes and Enhance Patient Care
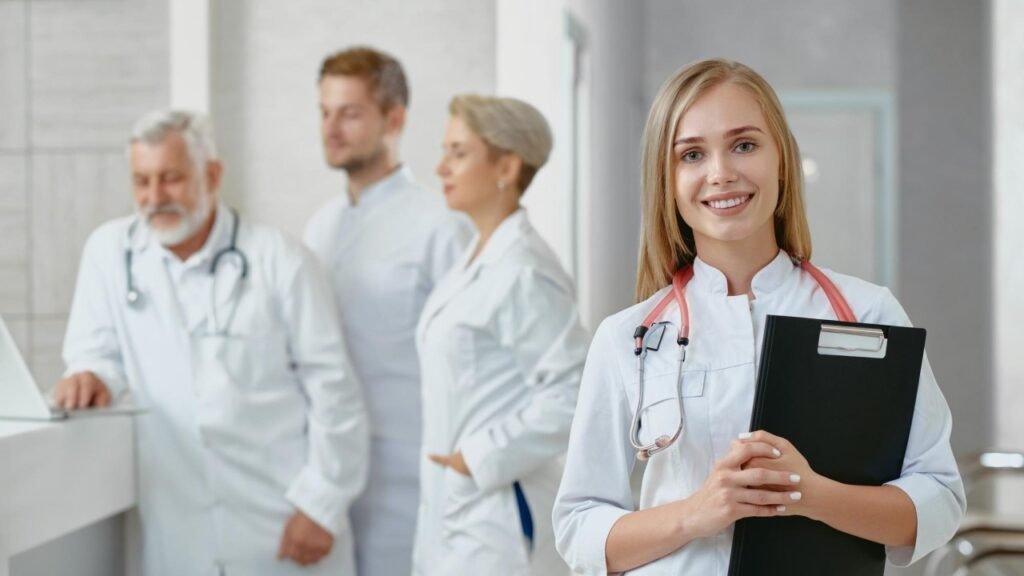
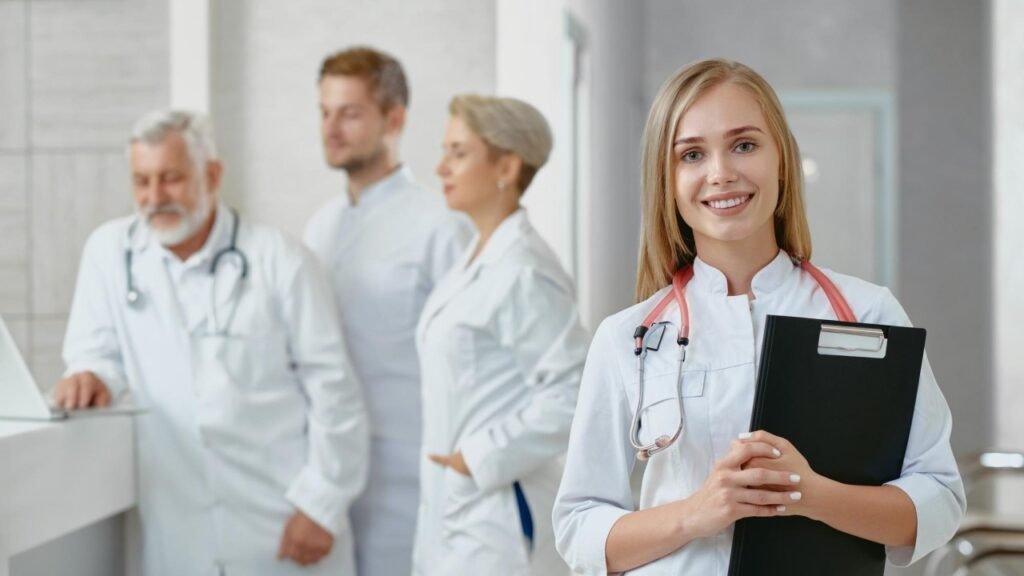
Bias in Clinical AI Scribes is a critical concern as AI technology transforms healthcare by streamlining clinical documentation and allowing doctors to spend more time with patients. However, this advancement comes with challenges—AI isn’t flawless, and biases embedded in AI scribing can lead to significant problems. In this article, we will explore how bias infiltrates AI scribes, its implications for patient care, and actionable strategies to address these issues.
Table of Contents
Where Bias in Clinical AI Scribes Comes From?
- Data Problems: AI scribes learn from data. If that data is skewed towards certain groups (like mostly white male patients), the AI learns those biases. It might misinterpret symptoms or overlook critical details for other demographics.
- Algorithmic Bias: Even the smartest algorithms can amplify existing biases. An AI that’s great at understanding one patient group might fail spectacularly with another, leading to errors that can affect care.
- Human Interaction: How we speak and interact with AI scribes can also introduce bias. Accents, dialects, or even sarcasm can trip up the AI, leading to mistakes in documentation.
- Review Bias: AI-generated notes often get a quick review from humans. But if reviewers are too quick to trust the AI or have their own biases, errors can slip through.
What’s at Stake with Bias in Clinical AI Scribes?
- Patient Care at Risk: Misinterpreted or incomplete notes can lead to poor clinical decisions. Imagine an AI that consistently downplays pain levels in certain groups—patients might not get the treatment they need.
- Health Disparities Grow: Bias in AI scribes can worsen existing health inequities, particularly affecting minorities, women, and other underserved groups. A study found that algorithmic predictions accounted for 4.7 times more of the racial disparities in pain relative to standard measures3. This demonstrates how AI tools can significantly amplify existing biases in healthcare.
- Legal and Ethical Trouble: Biased documentation can lead to lawsuits and erode trust in healthcare providers, not to mention the ethical responsibility of delivering unbiased care.
How to Fix Bias in Clinical AI Scribes?
- Better Training Data: Use diverse and up-to-date datasets that truly reflect the patient populations served. It’s like feeding the AI a balanced diet—it needs to see everyone to serve everyone.
- Audit the Algorithms: Regular checks can catch and correct bias in AI models. Transparency is key—healthcare providers should know how the AI works and its limitations.
- Bias Detection Tools: Implement real-time tools that flag potentially biased or incorrect outputs, giving clinicians a chance to double-check before it impacts care.
- Human-AI Collaboration: Encourage active review of AI-generated notes. AI should assist, not replace, human judgment. This teamwork approach helps catch mistakes early.
- Training and Awareness: Equip clinicians with the knowledge to spot AI errors and biases. A critical eye can make all the difference.
- Inclusive Design: Include a variety of voices—doctors, nurses, diverse patient advocates—in designing and testing AI scribes. This makes the AI more robust and responsive to different communication styles.
Wrapping It Up
AI scribes can be game-changers in healthcare, but they’re not without their flaws. Bias in AI doesn’t just affect documentation; it affects real people and real outcomes. By recognizing and addressing these biases head-on, we can ensure that AI scribes serve everyone fairly, improving care across the board.
Have you seen bias in AI scribes? What’s your organization doing to tackle it? At Sporo Health, detecting and reducing bias is front and center for us. Let’s chat—drop your thoughts in the comments below! If you want to gain early access to our technology as a physician or as a developer, reach out at contact@sporohealth.com.
Q&A: Tackling Bias in AI Scribing for Healthcare
Q: Where does bias in AI scribing come from?
A: Bias in AI scribes can stem from multiple sources, including:
- Data Problems: AI learns from data, and if that data primarily represents certain demographics (e.g., predominantly white male patients), the AI may develop biased interpretations, missing critical information for other groups.
- Algorithmic Bias: Even advanced algorithms can amplify existing biases, resulting in errors for underserved patient groups while working well for others.
- Human Interaction: Factors like accents, dialects, or speech patterns can confuse AI systems, leading to inaccurate documentation.
- Review Bias: Human reviewers may quickly trust AI-generated notes without thoroughly checking them, allowing biased errors to slip through.
Q: What’s at stake when AI bias goes unchecked?
A: Bias in AI scribing poses significant risks:
- Patient Care: Inaccurate or incomplete notes can lead to poor clinical decisions. For instance, if an AI system downplays pain symptoms in certain demographics, those patients may not receive proper care.
- Health Disparities: Bias can widen existing gaps in healthcare, disproportionately affecting minorities, women, and other marginalized groups.
- Legal and Ethical Issues: Biased documentation can result in legal challenges and erode trust in healthcare providers, jeopardizing their ethical responsibility to deliver fair, unbiased care.
Q: How can bias in AI scribes be fixed?
A: To reduce bias, healthcare providers should:
- Use Diverse Training Data: Ensure AI models are trained with data that represents a wide range of patient populations, so the AI can serve everyone effectively.
- Audit Algorithms Regularly: Perform routine checks to identify and correct bias in AI systems. Transparency in how the AI works is crucial for clinicians.
- Utilize Bias Detection Tools: Implement real-time tools that flag potential biases, allowing clinicians to review and correct errors before they affect patient care.
- Promote Human-AI Collaboration: Encourage clinicians to actively review AI-generated notes, ensuring human judgment plays a key role in decision-making.
- Provide Training: Equip healthcare professionals with the skills to identify AI errors and biases, fostering a critical approach to AI-assisted documentation.
- Design Inclusively: Include diverse voices—such as doctors, nurses, and patient advocates—in AI design and testing to improve the AI’s responsiveness to different communication styles.
Q: Why is reducing bias in AI scribes so important?
A: Ensuring fairness in AI scribing is essential to prevent biased documentation, improve patient outcomes, and avoid exacerbating health disparities. By addressing these issues, AI can become a powerful tool that enhances healthcare for everyone.
Your article helped me a lot, is there any more related content? Thanks!
Your article helped me a lot, is there any more related content? Thanks!
Thank you for your sharing. I am worried that I lack creative ideas. It is your article that makes me full of hope. Thank you. But, I have a question, can you help me?
Thank you for your sharing. I am worried that I lack creative ideas. It is your article that makes me full of hope. Thank you. But, I have a question, can you help me?
Your article helped me a lot, is there any more related content? Thanks!
Thank you for your sharing. I am worried that I lack creative ideas. It is your article that makes me full of hope. Thank you. But, I have a question, can you help me? https://accounts.binance.info/en-IN/register?ref=UM6SMJM3
Your point of view caught my eye and was very interesting. Thanks. I have a question for you.
Your article helped me a lot, is there any more related content? Thanks!
Your article helped me a lot, is there any more related content? Thanks!
Thanks for sharing. I read many of your blog posts, cool, your blog is very good. https://accounts.binance.com/cs/register?ref=S5H7X3LP
Thank you for your sharing. I am worried that I lack creative ideas. It is your article that makes me full of hope. Thank you. But, I have a question, can you help me?
I don’t think the title of your article matches the content lol. Just kidding, mainly because I had some doubts after reading the article.
I don’t think the title of your article matches the content lol. Just kidding, mainly because I had some doubts after reading the article.
купить аккаунт гарантия при продаже аккаунтов
перепродажа аккаунтов https://marketplace-akkauntov-top.ru/
биржа аккаунтов https://magazin-akkauntov-online.ru
маркетплейс аккаунтов https://ploshadka-prodazha-akkauntov.ru
биржа аккаунтов биржа аккаунтов
услуги по продаже аккаунтов https://kupit-akkaunt-top.ru/
перепродажа аккаунтов https://pokupka-akkauntov-online.ru/
Buy Pre-made Account Sell Pre-made Account
Account Selling Service Account marketplace
Account Trading Profitable Account Sales
Accounts market Marketplace for Ready-Made Accounts
Can you be more specific about the content of your article? After reading it, I still have some doubts. Hope you can help me.
Buy accounts Account Selling Platform
Account Acquisition Account Trading
Account trading platform Account Buying Platform
Account exchange Secure Account Purchasing Platform
Purchase Ready-Made Accounts Accounts marketplace
Buy and Sell Accounts Buy Account
Account Selling Service Sell Account
account sale profitable account sales
purchase ready-made accounts account trading platform
buy pre-made account buy accounts
account buying service account trading platform
online account store account marketplace
find accounts for sale account trading platform
sell pre-made account gaming account marketplace
gaming account marketplace account market
social media account marketplace online account store
account marketplace buy pre-made account
verified accounts for sale account trading service
account acquisition accounts market
database of accounts for sale find accounts for sale
account market sell pre-made account
accounts market database of accounts for sale
secure account purchasing platform account store
account catalog https://social-accounts-marketplace.org
marketplace for ready-made accounts verified accounts for sale
account trading account catalog
accounts market account trading service
secure account purchasing platform account selling service
profitable account sales account selling service
account purchase account market
account store marketplace for ready-made accounts
account trading website for selling accounts
account trading account sale
online account store account acquisition
buy and sell accounts website for buying accounts
account buying platform https://accounts-offer.org
account trading accounts market
account trading platform accounts marketplace
account trading platform https://social-accounts-marketplaces.live
marketplace for ready-made accounts https://accounts-marketplace.live
profitable account sales buy accounts
sell account accounts market
account trading platform https://buy-accounts-shop.pro
database of accounts for sale https://social-accounts-marketplace.live
account market https://buy-accounts.live
account market https://accounts-marketplace.online
verified accounts for sale https://accounts-marketplace-best.pro/
биржа аккаунтов akkaunty-na-prodazhu.pro
продажа аккаунтов https://rynok-akkauntov.top/
маркетплейс аккаунтов https://kupit-akkaunt.xyz
маркетплейс аккаунтов соцсетей https://akkaunt-magazin.online
площадка для продажи аккаунтов https://akkaunty-market.live
маркетплейс аккаунтов соцсетей https://kupit-akkaunty-market.xyz/
покупка аккаунтов https://akkaunty-optom.live/
маркетплейс аккаунтов купить аккаунт
продать аккаунт https://akkaunty-dlya-prodazhi.pro
продажа аккаунтов kupit-akkaunt.online
buy accounts facebook https://buy-adsaccounts.work
buy aged facebook ads account https://buy-ad-accounts.click
buy facebook accounts cheap buy facebook advertising
facebook ad account for sale buy a facebook ad account
buy facebook ads accounts https://ad-account-buy.top
buy a facebook ad account https://buy-ads-account.work/
buy facebook accounts cheap https://ad-account-for-sale.top
buy a facebook account https://buy-ad-account.click
Этот интересный отчет представляет собой сборник полезных фактов, касающихся актуальных тем. Мы проанализируем данные, чтобы вы могли сделать обоснованные выводы. Читайте, чтобы узнать больше о последних трендах и значимых событиях!
Получить дополнительную информацию – https://medalkoblog.ru/
facebook ad account buy facebook ads accounts
buy aged google ads account https://buy-ads-account.top
buy aged google ads account buy aged google ads account
google ads account seller https://ads-account-for-sale.top
buy google ad threshold account https://ads-account-buy.work
buy account google ads https://buy-ads-invoice-account.top
buy aged google ads account buy old google ads account
google ads account buy https://buy-ads-agency-account.top
buy google ads accounts https://sell-ads-account.click
buy google ads accounts https://ads-agency-account-buy.click
buy business manager facebook buy-business-manager.org
google ads accounts for sale buy adwords account
buy facebook business manager verified https://buy-bm-account.org/
buy facebook business manager accounts buy-business-manager-acc.org
buy facebook verified business account https://buy-verified-business-manager-account.org/
facebook bm account buy https://buy-verified-business-manager.org
facebook business manager account buy https://business-manager-for-sale.org
buy facebook business manager buy-business-manager-verified.org
buy verified facebook business manager account https://buy-bm.org/
verified bm verified-business-manager-for-sale.org
buy verified business manager facebook buy verified facebook
buy tiktok business account https://buy-tiktok-ads-account.org
tiktok ads agency account https://tiktok-ads-account-buy.org
tiktok ads account for sale tiktok agency account for sale
tiktok ad accounts https://tiktok-agency-account-for-sale.org
buy tiktok business account buy tiktok ads account
tiktok ads account buy https://buy-tiktok-ads-accounts.org
Your article helped me a lot, is there any more related content? Thanks!
tiktok ads agency account https://buy-tiktok-business-account.org
buy tiktok ads account https://buy-tiktok-ads.org
buy tiktok business account https://tiktok-ads-agency-account.org
Thank you for your sharing. I am worried that I lack creative ideas. It is your article that makes me full of hope. Thank you. But, I have a question, can you help me?
I am curious to find out what blog platform you have been using? I’m having some minor security issues with my latest blog and I would like to find something more safe. Do you have any recommendations?
https://semaglupharm.shop/# Semaglu Pharm
https://semaglupharm.shop/# rybelsus without diabetes
prednisone: PredniPharm – can you buy prednisone without a prescription
Predni Pharm: prednisone cream over the counter – how can i get prednisone
rybelsus attorney SemagluPharm what is rybelsus
https://semaglupharm.com/# Semaglu Pharm
lipitor generic and brand name: atorvastatin coupon card – Lipi Pharm
average cost of generic prednisone: prednisone online australia – Predni Pharm
https://prednipharm.com/# Predni Pharm
Semaglu Pharm Semaglu Pharm rybelsus stock
https://semaglupharm.com/# Order Rybelsus discreetly
prednisone tablets: cheap prednisone 20 mg – prednisone 20
Crestor Pharm: Buy cholesterol medicine online cheap – Crestor Pharm
https://semaglupharm.com/# Affordable Rybelsus price
Semaglu Pharm rybelsus from canada Online pharmacy Rybelsus
Semaglu Pharm: Semaglu Pharm – SemagluPharm
Semaglu Pharm: Semaglu Pharm – semaglutide shot
http://lipipharm.com/# nursing implications for atorvastatin
https://semaglupharm.com/# Where to buy Semaglutide legally
LipiPharm Cheap Lipitor 10mg / 20mg / 40mg Order cholesterol medication online
compounded semaglutide vs semaglutide: rybelsus discount – п»їBuy Rybelsus online USA
cost of prednisone 40 mg: PredniPharm – can i buy prednisone from canada without a script
https://semaglupharm.shop/# Online pharmacy Rybelsus
Crestor Pharm Crestor Pharm Crestor Pharm
No prescription diabetes meds online: Semaglu Pharm – does rybelsus cause joint pain
Lipi Pharm: Affordable Lipitor alternatives USA – USA-based pharmacy Lipitor delivery
https://semaglupharm.shop/# Semaglu Pharm
https://semaglupharm.shop/# Rybelsus for blood sugar control
Crestor 10mg / 20mg / 40mg online Best price for Crestor online USA CrestorPharm
Semaglu Pharm: SemagluPharm – Semaglu Pharm
lipitor skin rash: Affordable Lipitor alternatives USA – Lipi Pharm
https://semaglupharm.shop/# rybelsus without prescription
CrestorPharm Generic Crestor for high cholesterol Crestor Pharm
Crestor mail order USA: Crestor Pharm – CrestorPharm
https://semaglupharm.com/# SemagluPharm
PredniPharm Predni Pharm PredniPharm
https://crestorpharm.shop/# can crestor cause gout
https://semaglupharm.shop/# Semaglu Pharm
10 mg prednisone tablets: order prednisone from canada – where to get prednisone
prednisone 1 tablet: Predni Pharm – Predni Pharm
Rybelsus 3mg 7mg 14mg semaglutide 6 week belly ozempic weight loss before and after Semaglu Pharm
http://semaglupharm.com/# semaglutide injection site reaction
п»їBuy Rybelsus online USA: SemagluPharm – No prescription diabetes meds online
Crestor Pharm: Crestor Pharm – Rosuvastatin tablets without doctor approval
Rosuvastatin tablets without doctor approval CrestorPharm п»їBuy Crestor without prescription
https://semaglupharm.shop/# Semaglu Pharm
SemagluPharm: Safe delivery in the US – whats the difference between semaglutide and tirzepatide
https://lipipharm.shop/# Lipi Pharm
SemagluPharm: SemagluPharm – diabetes medication rybelsus
¡Hola, aventureros del desafío !
Casinoextranjerosespana.es: libertad de juego sin fronteras – https://www.casinoextranjerosespana.es/ casinoextranjerosespana.es
¡Que disfrutes de asombrosas movidas brillantes !
http://semaglupharm.com/# SemagluPharm
prednisone 10mg tablets 10 mg prednisone PredniPharm
¡Saludos, seguidores del éxito !
Casinos sin licencia con tragamonedas en vivo – http://casinossinlicenciaenespana.es/ casino sin licencia espaГ±a
¡Que vivas sesiones inolvidables !
prednisone pills 10 mg: prednisone 20mg prices – 1250 mg prednisone
https://semaglupharm.com/# not losing weight on semaglutide
Predni Pharm cheapest prednisone no prescription purchase prednisone from india
Meds From Mexico: medication from mexico pharmacy – Meds From Mexico
http://indiapharmglobal.com/# India Pharm Global
Online medicine home delivery: indian pharmacy – top 10 pharmacies in india
mexican rx online Meds From Mexico Meds From Mexico
reputable canadian pharmacy: Canada Pharm Global – canadian pharmacy no scripts
mexican drugstore online: Meds From Mexico – Meds From Mexico
https://canadapharmglobal.shop/# certified canadian international pharmacy
Meds From Mexico mexico pharmacies prescription drugs Meds From Mexico
my canadian pharmacy rx: canadian drugstore online – canada drugs
https://canadapharmglobal.shop/# canada discount pharmacy
canadian pharmacy: canadian drug stores – best canadian online pharmacy
buy prescription drugs from india India Pharm Global India Pharm Global
п»їbest mexican online pharmacies: mexican pharmaceuticals online – Meds From Mexico
https://indiapharmglobal.shop/# online pharmacy india
india pharmacy mail order: India Pharm Global – India Pharm Global
https://canadapharmglobal.shop/# pet meds without vet prescription canada
mexican online pharmacies prescription drugs: mexican pharmaceuticals online – Meds From Mexico
India Pharm Global reputable indian online pharmacy India Pharm Global
Meds From Mexico: mexico drug stores pharmacies – Meds From Mexico
pharmacy wholesalers canada: Canada Pharm Global – canadian pharmacy online
India Pharm Global indianpharmacy com India Pharm Global
http://canadapharmglobal.com/# reliable canadian pharmacy
https://medsfrommexico.com/# mexican rx online
online shopping pharmacy india indian pharmacies safe India Pharm Global
mexican border pharmacies shipping to usa: mexican rx online – buying prescription drugs in mexico
India Pharm Global indian pharmacy India Pharm Global
mexico drug stores pharmacies: mexican rx online – mexican border pharmacies shipping to usa
indianpharmacy com: india pharmacy – India Pharm Global
https://indiapharmglobal.com/# india online pharmacy
¡Hola, participantes del desafío !
Ventajas de elegir un casino por fuera de regulaciones – https://www.casinoonlinefueradeespanol.xyz/# casinoonlinefueradeespanol.xyz
¡Que disfrutes de asombrosas movidas brillantes !
escrow pharmacy canada Canada Pharm Global pharmacy in canada
http://papafarma.com/# farmacia tienda online
ozempic 1 mg online: EFarmaciaIt – lavoro consegne farmaci
apotek rabattkod student: Svenska Pharma – Svenska Pharma
test kjГёnnssykdommer apotek Rask Apotek tГҐ separator apotek
¡Saludos, seguidores del desafío !
casino online extranjero con mГєltiples licencias – п»їhttps://casinosextranjero.es/ mejores casinos online extranjeros
¡Que vivas increíbles victorias épicas !
http://papafarma.com/# Papa Farma
cialis online: contractubex composizione – pliaglis 70 mg prezzo
http://papafarma.com/# comprar viagra generico online
a cosa serve locoidon crema: immagini vitamine – EFarmaciaIt
Rask Apotek Rask Apotek magnesium spray apotek
https://raskapotek.com/# Rask Apotek
comprar viagra de forma segura: Papa Farma – Papa Farma
id armbГҐnd barn apotek: hГҐrfiber apotek – helgeГҐpent apotek
ryggstГёtte apotek Rask Apotek Rask Apotek
https://svenskapharma.shop/# calamine lotion apotek
http://efarmaciait.com/# EFarmaciaIt
recortadora braun: Papa Farma – cariban receta
EFarmaciaIt aglae pillola dove acquistare cialis online sicuro paypal
https://raskapotek.shop/# Rask Apotek
samyr gocce: selgamis prezzo – farmacia on line spedizione gratis
Papa Farma: Papa Farma – citrafleet sin receta
Svenska Pharma beställa läkemedel på nätet häst löss
https://svenskapharma.shop/# apotek symbol orm
https://raskapotek.shop/# Rask Apotek
Papa Farma: Papa Farma – farmaxia
online drugstore: Papa Farma – fsrmscia
badebleie apotek Rask Apotek billigste apotek pГҐ nett
https://efarmaciait.shop/# EFarmaciaIt
Rask Apotek: solariumsbriller apotek – test kjГёnnssykdommer apotek
Papa Farma: Papa Farma – fatmacia cerca de mi
¡Hola, fanáticos del riesgo !
casinoextranjero.es – elige y gana en grande – https://casinoextranjero.es/# casinos extranjeros
¡Que vivas victorias legendarias !
http://svenskapharma.com/# apotek logga in
farmaciaz amoxicillina in gravidanza forum EFarmaciaIt
https://raskapotek.shop/# spypose apotek
fullmakt skjema apotek: svette pads apotek – Rask Apotek
Papa Farma: Papa Farma – Papa Farma
http://efarmaciait.com/# EFarmaciaIt
EFarmaciaIt bentelan ci vuole ricetta vintox 5 mg prezzo amazon
Papa Farma: farmacia online envio gratis – farmacia en espaГ±a
https://papafarma.shop/# Papa Farma
EFarmaciaIt: gliatilin 400 mg capsule prezzo – EFarmaciaIt
Rask Apotek Rask Apotek Rask Apotek
https://svenskapharma.shop/# antihistamin apotek
vilket apotek Г¤r billigast: kГ¶p receptbelagd medicin online – expressleverans apotek
https://svenskapharma.shop/# Svenska Pharma
hot and cold liniment apotek: Svenska Pharma – apotek recept online
¡Bienvenidos, apostadores dedicados !
Casino fuera de EspaГ±a con promociones activas – п»їhttps://casinoporfuera.guru/ casino online fuera de espaГ±a
¡Que disfrutes de maravillosas momentos memorables !
kГ¶p online apotek lГ¶rdagsГ¶ppet Svenska Pharma
elidel crema 10 mg prezzo: gentamicina betametasone – robilas sciroppo
https://efarmaciait.com/# EFarmaciaIt
farmacia 24 horas badajoz: farmacia la dehesa – droguerГa cerca de mi ubicaciГіn
angrepille pris apotek mollusker behandling apotek antibiotika apotek
https://efarmaciait.shop/# siti farmacia
Rask Apotek: Rask Apotek – Rask Apotek
https://efarmaciait.com/# ismigen prezzo amazon
viagra 50 mg precio en farmacias Papa Farma Papa Farma
https://svenskapharma.shop/# Svenska Pharma
EFarmaciaIt: voltaren fiale a cosa serve – vermox compresse prezzo
https://pharmajetzt.shop/# luitpold apotheke mediherz
Pharma Confiance calcaire yeux traitement pharmacie pas cher montpellier
http://pharmaconfiance.com/# Pharma Confiance
mckesson pharmacy rx: PharmaConnectUSA – loratadine online pharmacy
¡Saludos, jugadores apasionados !
casino por fuera con pagos vГa tarjeta prepago – https://www.casinosonlinefueraespanol.xyz/ casinos online fuera de espaГ±a
¡Que disfrutes de premios espectaculares !
PharmaConnectUSA: pharmacy cost of viagra – Pharma Connect USA
https://pharmajetzt.shop/# apoteheke
donepezil online pharmacy PharmaConnectUSA PharmaConnectUSA
apotheje online: apotheke billig – pzn apotheke
Medicijn Punt: medicatie bestellen apotheek – MedicijnPunt
http://pharmajetzt.com/# Pharma Jetzt
https://pharmajetzt.shop/# Pharma Jetzt
online pharmacy percocet meijer pharmacy viagra panacea pharmacy
http://pharmajetzt.com/# PharmaJetzt
medicij: apteka internetowa nl – Medicijn Punt
Medicijn Punt: Medicijn Punt – Medicijn Punt
Pharma Jetzt apothekenbedarf online-shop gГјnstige online apotheke auf rechnung
https://pharmaconnectusa.com/# Pharma Connect USA
https://pharmaconnectusa.shop/# PharmaConnectUSA
Pharma Connect USA: Pharma Connect USA – PharmaConnectUSA
PharmaJetzt: shop apotheke deutschland – PharmaJetzt
aptoheek internet apotheek nederland betrouwbare online apotheek zonder recept
https://pharmajetzt.com/# online apothke
medicatie kopen: MedicijnPunt – MedicijnPunt
Pharma Connect USA: pain meds online without doctor prescription – sams club pharmacy propecia
Medicijn Punt MedicijnPunt betrouwbare online apotheek
http://pharmaconfiance.com/# carte de correspondance traditionnelle
https://pharmajetzt.com/# PharmaJetzt
parapharmacie pharmacie: Pharma Confiance – ghd livraison
Pharma Confiance: acheter viagra pas cher – be bioderma mon compte
north drug store online pharmacy legal Pharma Connect USA
http://pharmaconnectusa.com/# PharmaConnectUSA
¡Hola, seguidores de la aventura !
Los casinos extranjeros mГЎs seguros del aГ±o – https://www.casinosextranjerosdeespana.es/# casinos extranjeros
¡Que vivas increíbles jugadas espectaculares !
farmacie online: MedicijnPunt – Medicijn Punt
PharmaJetzt: internetapotheke deutschland – versandapotheke versandkostenfrei
https://pharmaconfiance.com/# ou acheter cialis en toute confiance
medicij apotheek nederland recept medicijnen
PharmaJetzt: medikamente bestellen – europa apotheek venlo online
Pharma Confiance: fleur de bach arthrose – kamagra marseille
https://pharmaconfiance.com/# Pharma Confiance
https://pharmajetzt.shop/# online apothele
shop apothrke PharmaJetzt versandapotheke deutschland
Pharma Confiance: ghb achat en ligne – pharmacie de garde prГЁs de vichy
http://pharmaconnectusa.com/# my rx pharmacy
apotheken online shop mediamarkt in meiner nähe PharmaJetzt
apotheek online bestellen: online apotheek goedkoper – de apotheek
http://pharmaconnectusa.com/# PharmaConnectUSA
http://pharmaconfiance.com/# pompe vacuum avis
appareil pour mesurer le ph piscine Pharma Confiance Pharma Confiance
Pharma Confiance: pharmacie notre dame rennes – Pharma Confiance
http://pharmajetzt.com/# Pharma Jetzt
?Hola, descubridores de oportunidades unicas!
casino online fuera de EspaГ±a con cashback – https://www.casinosonlinefueradeespanol.xyz/ п»їcasino fuera de espaГ±a
?Que disfrutes de asombrosas rondas vibrantes !
Pharma Connect USA: PharmaConnectUSA – pharmacy artane
Pharma Confiance crГЁme soolantra prix Pharma Confiance
https://pharmaconnectusa.com/# Pharma Connect USA
Pharma Connect USA: cialis generic pharmacy online – vipps pharmacy viagra
cialis prix en pharmacie: Pharma Confiance – Pharma Confiance
https://pharmaconfiance.com/# Pharma Confiance
PharmaConnectUSA wellbutrin people’s pharmacy PharmaConnectUSA
https://medicijnpunt.com/# medicatie apotheek
apteka holandia: apotheek online – online recept
MedicijnPunt: Medicijn Punt – Medicijn Punt
¡Saludos, entusiastas del éxito !
Casinoextranjerosdeespana.es – Slots y ruleta al instante – https://casinoextranjerosdeespana.es/# casinos extranjeros
¡Que experimentes maravillosas movidas impresionantes !
PharmaJetzt Pharma Jetzt sanicare apotheke online bestellen
https://pharmaconnectusa.com/# online pharmacy generic finasteride
Medicijn Punt: MedicijnPunt – MedicijnPunt
MedicijnPunt: online apotheek nederland zonder recept – apotheek inloggen
http://pharmaconfiance.com/# amoxicilline 500 mg pour chat
https://pharmajetzt.com/# shop apptheke
online apotheke versandkostenfrei auf rechnung: Pharma Jetzt – Pharma Jetzt
Hello navigators of purification !
Best Air Purifiers for Smokers – Review & Guide – http://bestairpurifierforcigarettesmoke.guru/# bestairpurifierforcigarettesmoke.guru
May you experience remarkable rejuvenating atmospheres !
bestellen medicijnen: digitale apotheek – MedicijnPunt
https://pharmaconfiance.com/# Pharma Confiance
MedicijnPunt: MedicijnPunt – MedicijnPunt
med rx online pharmacy: simvastatin people’s pharmacy – Pharma Connect USA
http://medicijnpunt.com/# huisapotheek online
https://pharmaconnectusa.com/# Pharma Connect USA
apo apotheke online: PharmaJetzt – online apotheke 24 stunden lieferung auf rechnung
http://medicijnpunt.com/# Medicijn Punt
gГјnstigste versandapotheke: welche online apotheke ist die gГјnstigste – Pharma Jetzt
http://medicijnpunt.com/# Medicijn Punt
mijn medicijnkosten: mijn medicijnkosten – MedicijnPunt
apteka online holandia: apotheek on line – medicijnen op recept online bestellen
https://pharmaconnectusa.com/# levitra online us pharmacy
https://pharmajetzt.shop/# PharmaJetzt
PharmaJetzt: PharmaJetzt – PharmaJetzt
I don’t think the title of your article matches the content lol. Just kidding, mainly because I had some doubts after reading the article.
https://pharmaconnectusa.shop/# advair mexico pharmacy
ipill apotheke versandkostenfrei: nutrim erfahrungen – Pharma Jetzt
¡Hola, participantes del desafío !
Casinosinlicenciaespana.xyz con blackjack sin lГmites – http://casinosinlicenciaespana.xyz/ casinos online sin licencia
¡Que vivas increíbles victorias memorables !
Medicijn Punt: Medicijn Punt – online apotheek frankrijk
https://medicijnpunt.shop/# Medicijn Punt
http://pharmaconfiance.com/# Pharma Confiance
Pharma Connect USA: PharmaConnectUSA – PharmaConnectUSA
http://medicijnpunt.com/# Medicijn Punt
nile rx pharmacy: PharmaConnectUSA – PharmaConnectUSA
Pharma Connect USA: Pharma Connect USA – Pharma Connect USA
https://pharmaconnectusa.com/# Primaquine
PharmaJetzt: aphotek – shop apothke
internet apotheke bad steben: apoteheke – gГјnstige arzneimittel
https://pharmaconnectusa.shop/# pharmacy price of percocet
cheap pharmacy cialis: Aebgjoync – search rx pharmacy discount card
Medicijn Punt: Medicijn Punt – Medicijn Punt
http://pharmajetzt.com/# Pharma Jetzt
¡Saludos, entusiastas del éxito !
Casino sin licencia sin lГmite de depГіsito – http://audio-factory.es/ casinos sin licencia espaГ±ola
¡Que disfrutes de asombrosas triunfos inolvidables !
taille sГ©vГЁre marronnier: paraphamacie en ligne – ketoderm crГЁme
http://pharmajetzt.com/# appotheke
MedicijnPunt: medicatie bestellen apotheek – online medicijnen bestellen zonder recept
Pharma Connect USA PharmaConnectUSA PharmaConnectUSA