The Intersection of AI in Clinical Notes
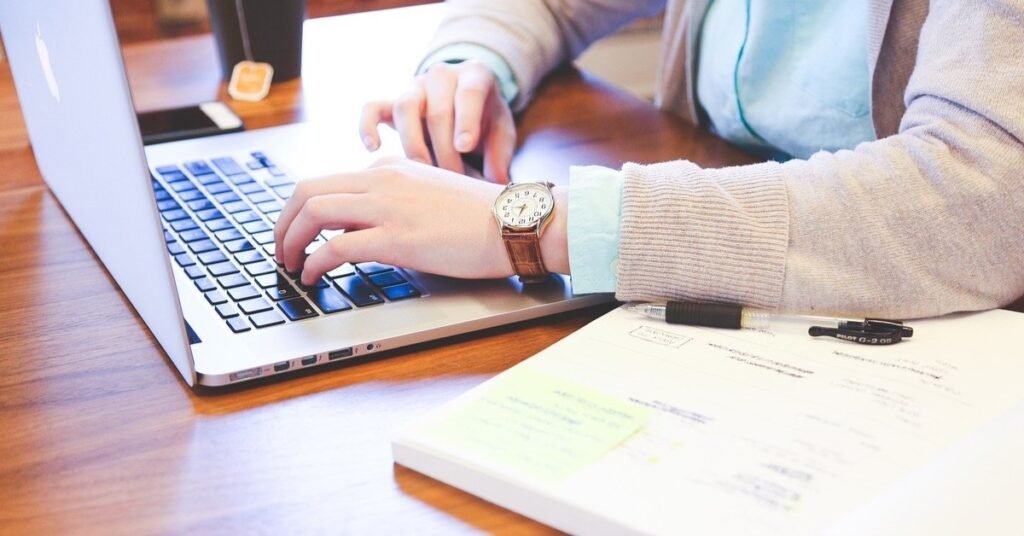
Language Models (LLMs) are revolutionizing the healthcare industry by their application in clinical summarization of chart notes. Chart notes, or medical records, are detailed documents that contain the minutiae of a patient’s medical history, diagnoses, treatment plans, lab work, and progress notes. Traditionally, the summarization of these notes has been a time-consuming and labor-intensive process for healthcare providers, often diverting their attention from patient care to Electronic Health Record (EHR) work. The advent of LLM in healthcare is changing this narrative by automating many portions of the patient visitation process – one of the critical components being manual chart review (MCR).
LLMs leverage advanced natural language processing (NLP) techniques to understand and interpret the decentralized, often disorganized libraries of complex, jargon-heavy medical documentation. Sporo takes advantage of this by training on vast datasets of medical records, to generate concise, accurate “medical synopses,” each tailored to the physician’s needs. This capability not only saves healthcare professionals’ time but also minimizes human error, leading to improved patient outcomes. Moreover, these summaries can be customized to the needs of various stakeholders, such as physicians who need quick overviews or specialists who require detailed analyses of specific conditions.
Furthermore, the application of LLMs in clinical summarization supports the integration of patient data across different healthcare systems, facilitating a more holistic approach to patient care. By providing quick access to patient histories, LLMs assist in identifying trends, potential health risks, and opportunities for preventive measures or even diagnostics, thereby contributing to more informed decision-making.
Recent work has detailed the architecture and components of multimodal LLMs, and Sporo leverages the cutting-edge technical advancements of 2023 and 2024 into its product. Multimodal LLMs are designed to handle inputs from diverse modalities (e.g., images, audio, 3D) and produce outputs in various forms. The architecture includes a modality encoder, input and output projectors, and a modality generator, with a focus on lightweight components for efficient and cost-effective training and usage. These multimodal LLMs can enhance tasks like semantic understanding, reasoning, and decision-making across all stages of patient health information. Combined with the decentralized but overflowing quantities of medical data available both to the public and private Large Academic Medical Centers (LAMCs), the potentials are endless for the usage of LLMs in clinical workflow and in diagnostics.
Many argue that the implementation of LLMs in clinical settings also raises concerns regarding data privacy and security. It’s essential to ensure that these technologies comply with healthcare regulations like HIPAA in the US, safeguarding patient information. Here at Sporo, we face these challenges head on. Our vision for our gen-AI technology in healthcare is undeniably marking a significant step towards more efficient, patient-centered care.